At Rhino Health, we transform healthcare AI by integrating Edge Computing and Federated Learning into a cohesive Federated Computing strategy. This innovative approach provides AI developers with swift and secure access to healthcare data, dramatically reducing setup times from months to days and ensuring data privacy across global networks.
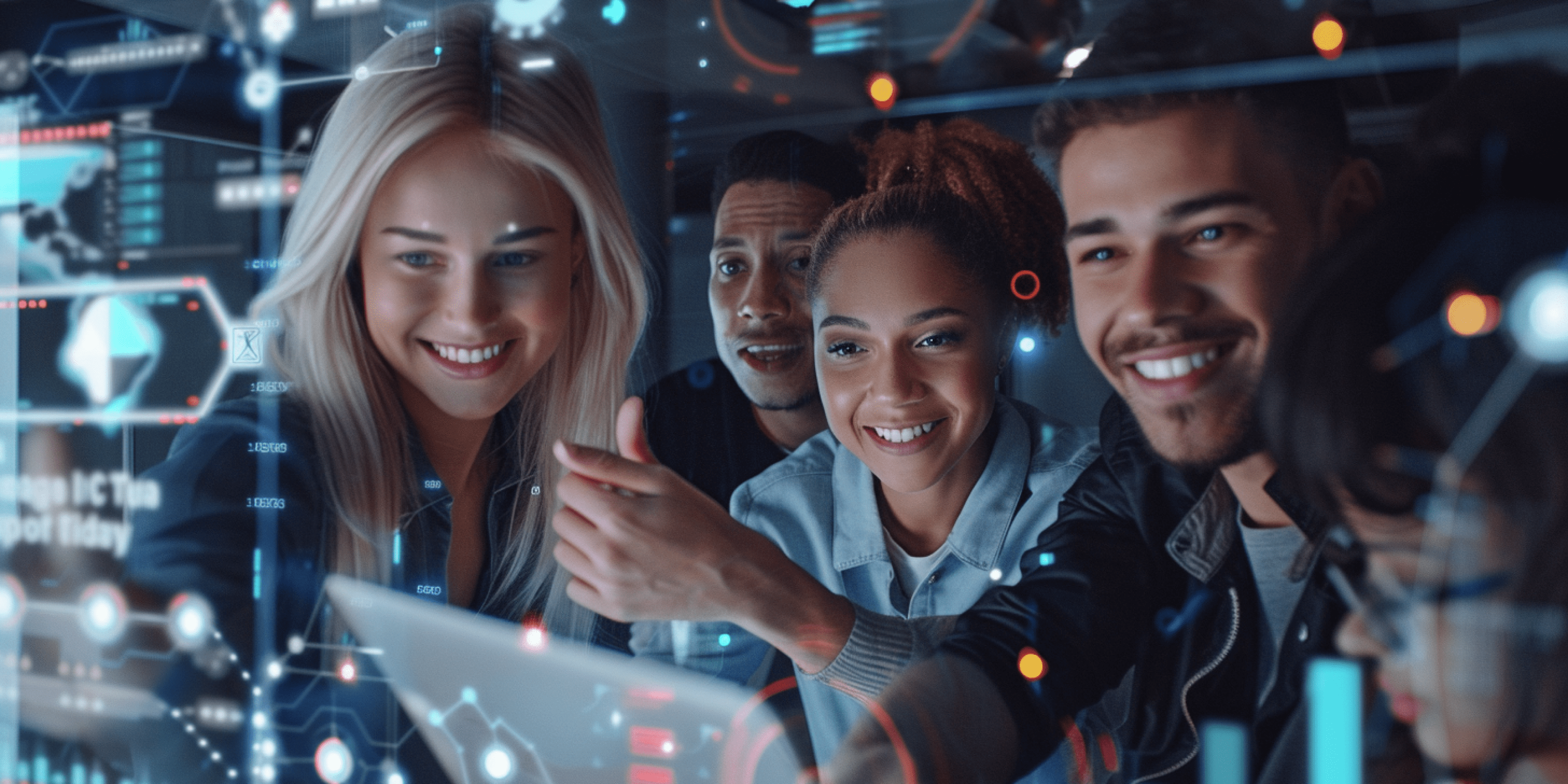
The Backbone of Modern Healthcare AI
Federated Computing allows working with data across multiple sites while keeping that data at rest behind each site’s firewall. This technique enables multiple entities to contribute to AI model training without disclosing raw data, safeguarding the privacy of each dataset. Our Federated Computing strategy ensures data integrity and compliance while minimizing latency and maximizing efficiency, making it an essential tool for advancing global healthcare solutions.
With the Federated Computing Platform, AI innovators find an unmatched environment for:
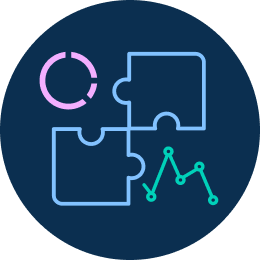

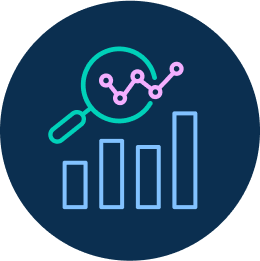


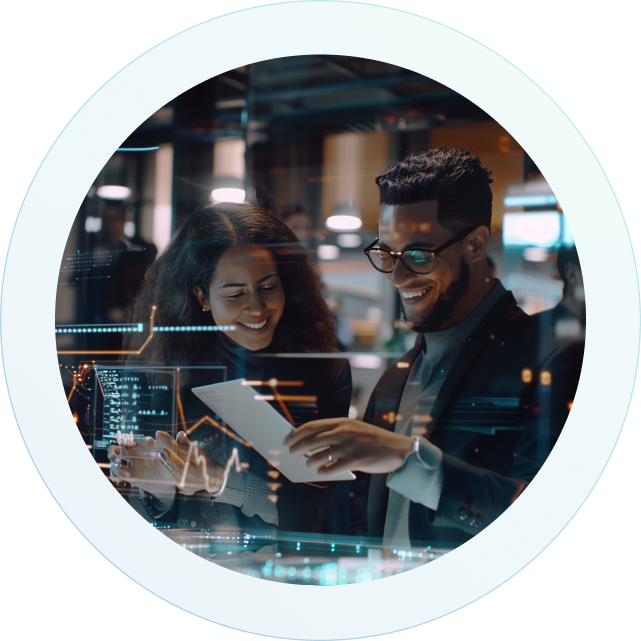
.jpg)
Why choose Rhino Health?
Federated Computing is the best way to collaborate on healthcare data
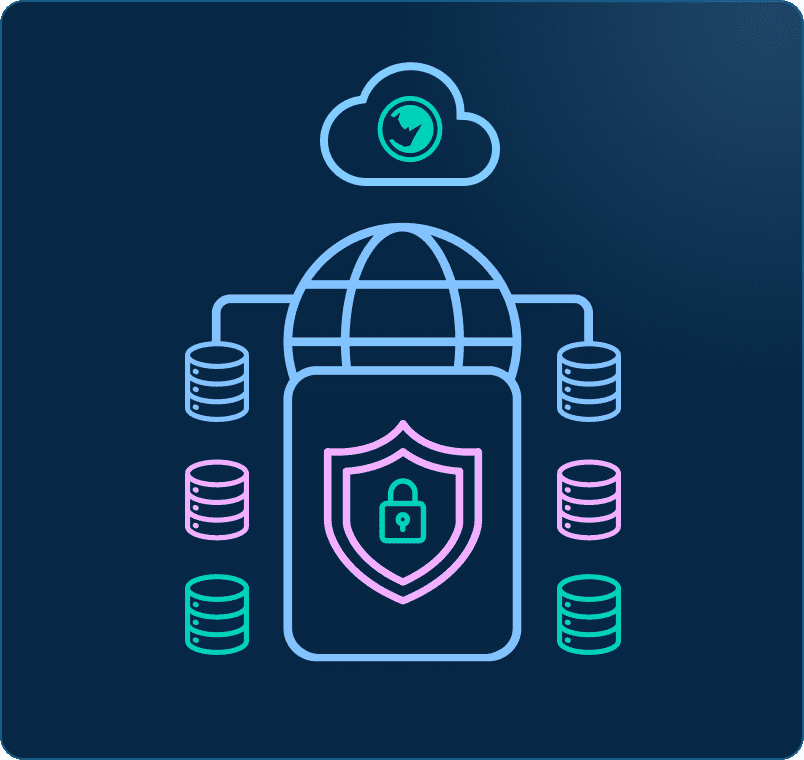
Data sovereignty
Data custodians maintain full control over their data by keeping it within their network. This reduces regulatory hurdles associated with cross-jurisdiction data transfer and makes it easier to maintain full data lineage.
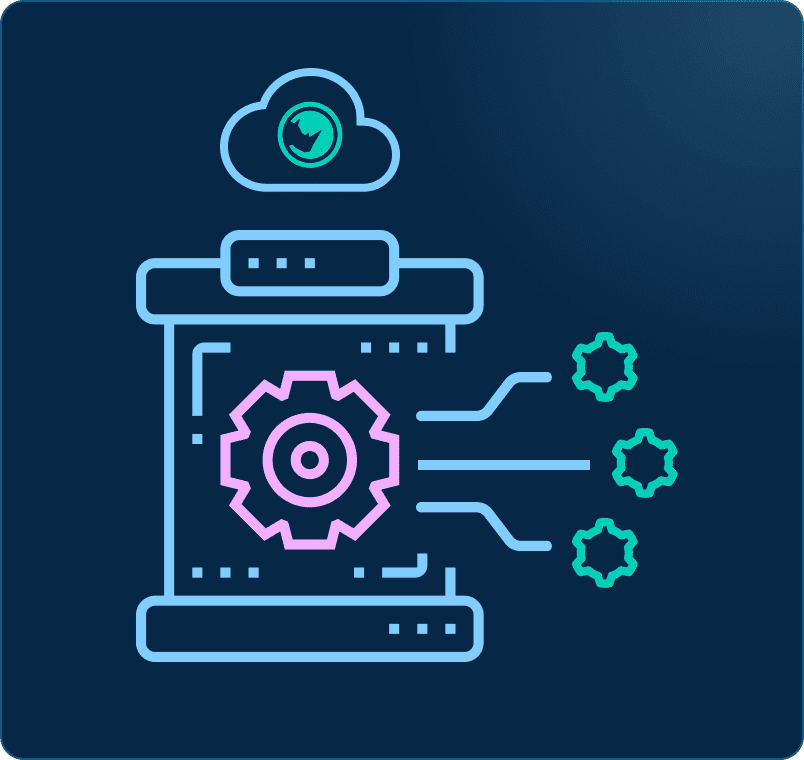
Operational efficiency
Reduce the data engineering and IT burden for data custodians and accelerate the completion of projects through central orchestration of projects with processing at the edge.
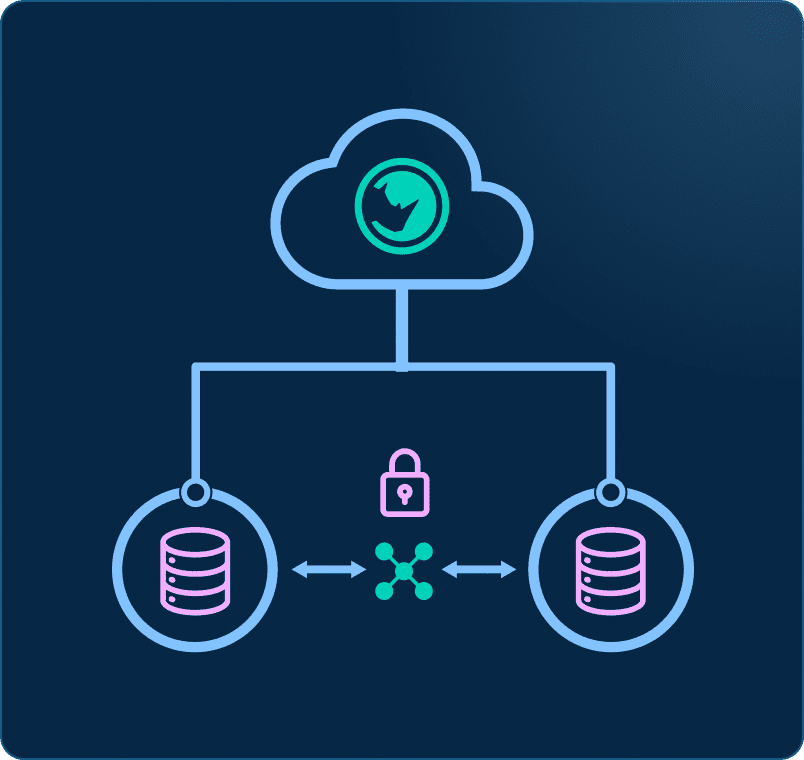
Diverse datasets
Leverage a broader range of high-quality, diverse datasets from multiple institutions, enhancing the robustness and generalizability of your AI models through federated collaboration.
Discover the power of Rhino Health’s Federated Computing Platform

Unlock insights with ease
Explore the full potential of data with our Federated Datasets—your gateway to seamless multi-site analytics.
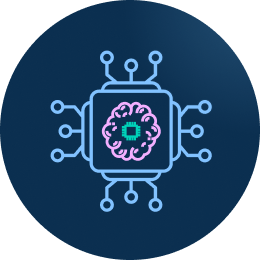
Centralize your innovation workflow
Streamline your AI journey from development to deployment with a unified platform, navigating the complexities of distributed data with unmatched simplicity.
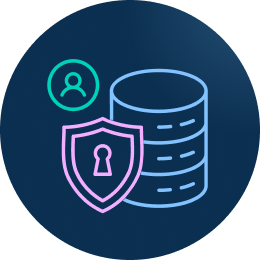
Compliance without compromise
Embrace innovation while adhering to rigorous security and privacy standards, including ISO 27001, SOC 2, HIPAA, and GDPR compliance. Your data remains secure under the vigilant protection of data custodians, allowing you to focus on driving impactful change.
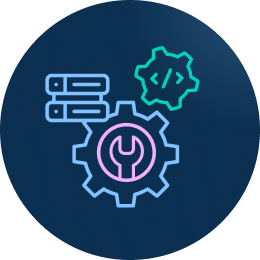
Tools tailored for trailblazers
Elevate your team’s productivity with groundbreaking tools like our Harmonization Copilot and Federated Datasets, designed for the needs of data engineers and scientists.

Rhino Health has built the largest global federated network of hospitals. Join over 50 leading global institutions in the US, EMEA, and APAC that are reshaping healthcare & life sciences with Rhino Health. Innovators from around the world are making their data available for collaboration via our Federated Datasets and using the Federated Computing Platform to complete a range of research projects across data modalities and disciplines.
Featured Insights & Highlights
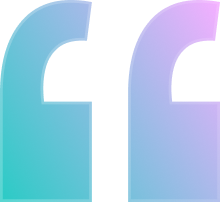
Rhino Health's Federated Datasets feature enables Assuta to collaborate with industry partners to promote healthcare innovation while ensuring patient privacy is held to the highest standard. We have been able to accelerate collaborations from months to weeks.”
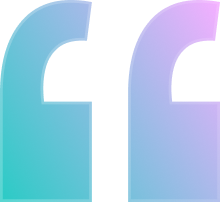
Federated Datasets help builders like me quickly and efficiently search for available datasets in order to deploy and validate new AI solutions while preserving privacy and compliance.”
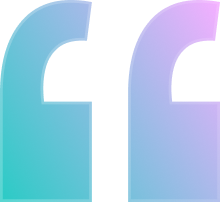
As a model developer, I always need more Real-world Data to validate and refine my AI models. Federated Datasets help me search for and obtain access to relevant datasets to promote my research.”
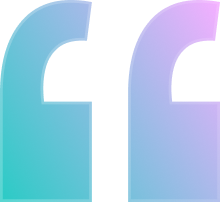
Rhino Health's Federated Computing Platform provided us with the secure and robust infrastructure needed to test our Quantile Regression AI model in a Federated way. With their support, we were able to simulate the development of predictive models for the care of our premature newborn patients based on data from different hospitals. Rhino Health's expertise in Edge Computing and Federated Learning streamlined our workflow and fortified the privacy and security of our patient data, which is paramount in our field. We look forward to continuing this fruitful partnership as we explore new frontiers in healthcare AI.”
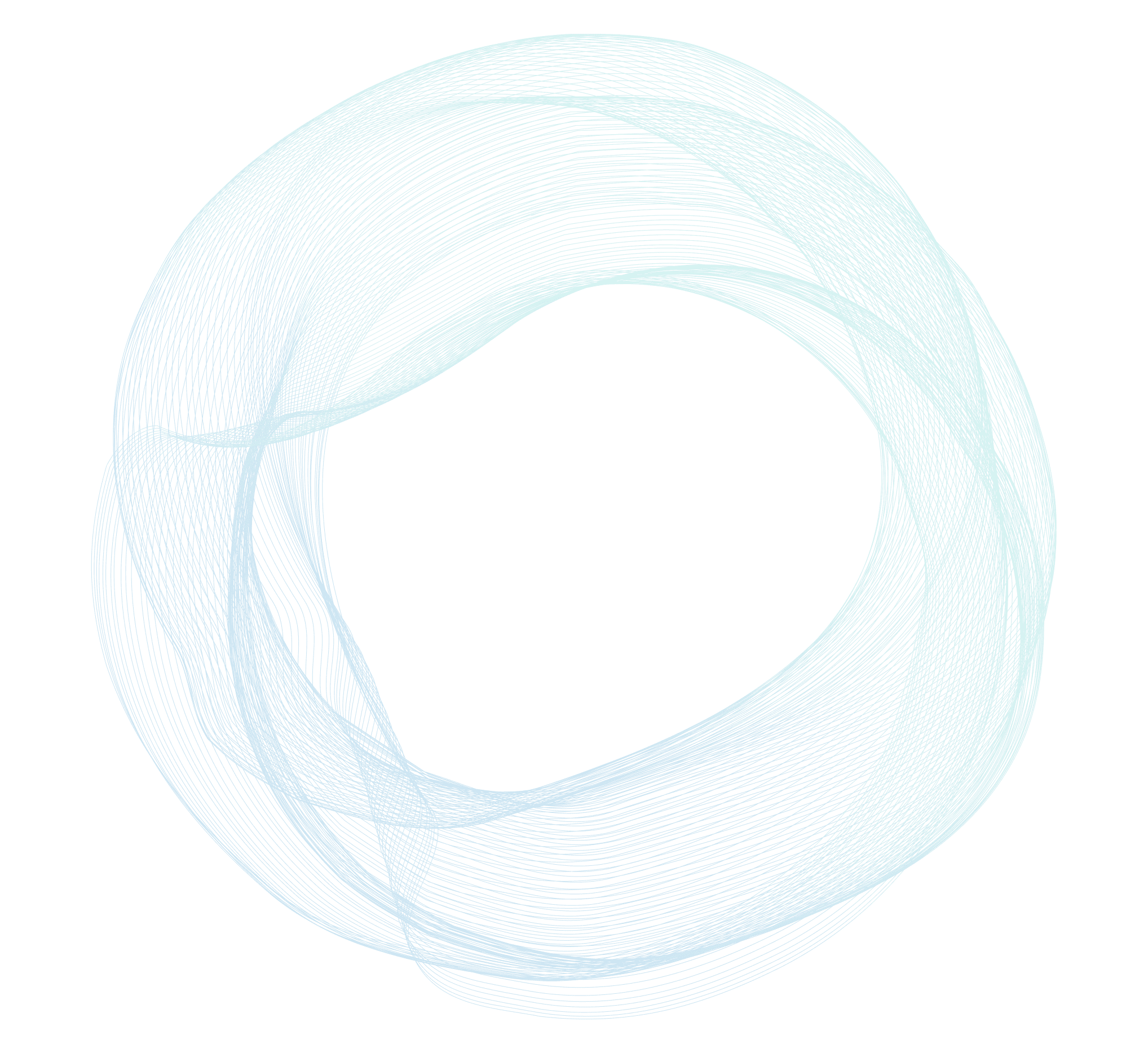
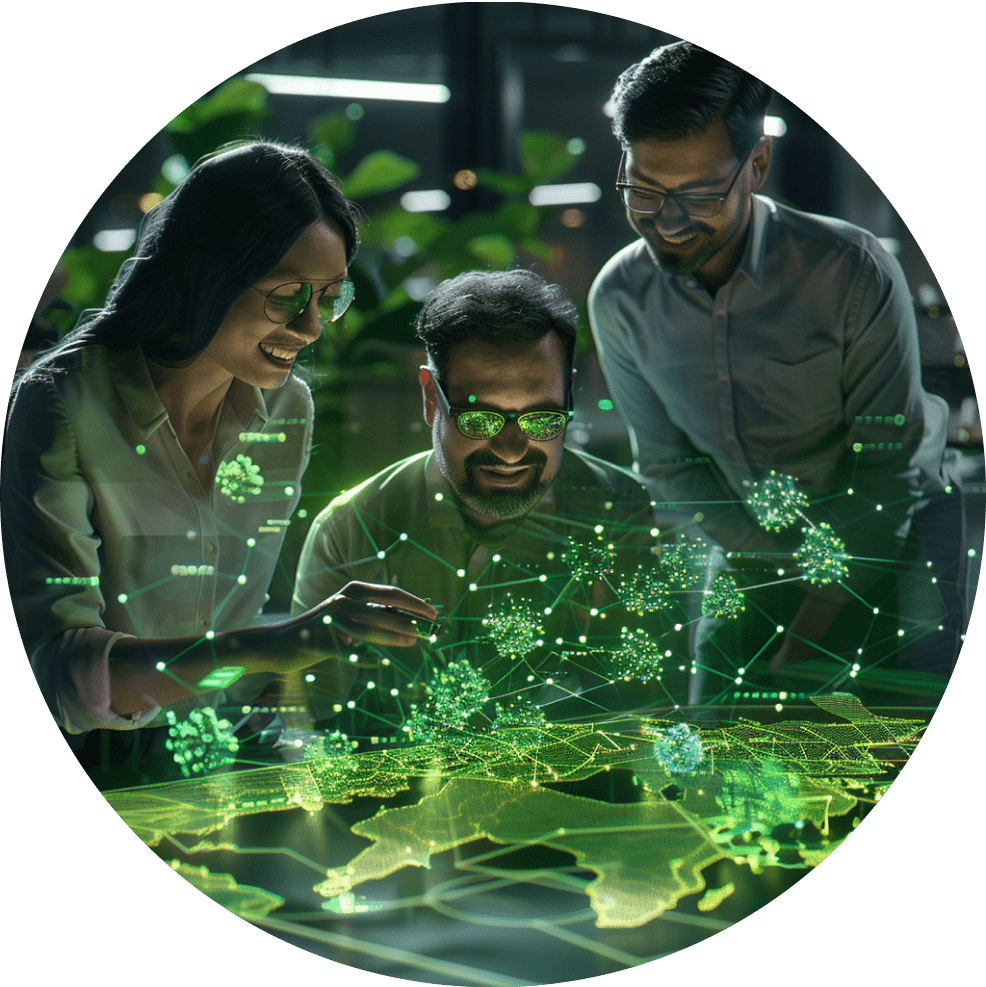
Ready to see how our Federated Computing Platform can transform healthcare? Join the Federated Network and start working with our collaborators’ data today!
Rhino Health is your partner in data collaborations. Join our Community, connect with us on LinkedIn, and stay updated with our Newsletter. Let’s collectively forge a healthier world powered by data.