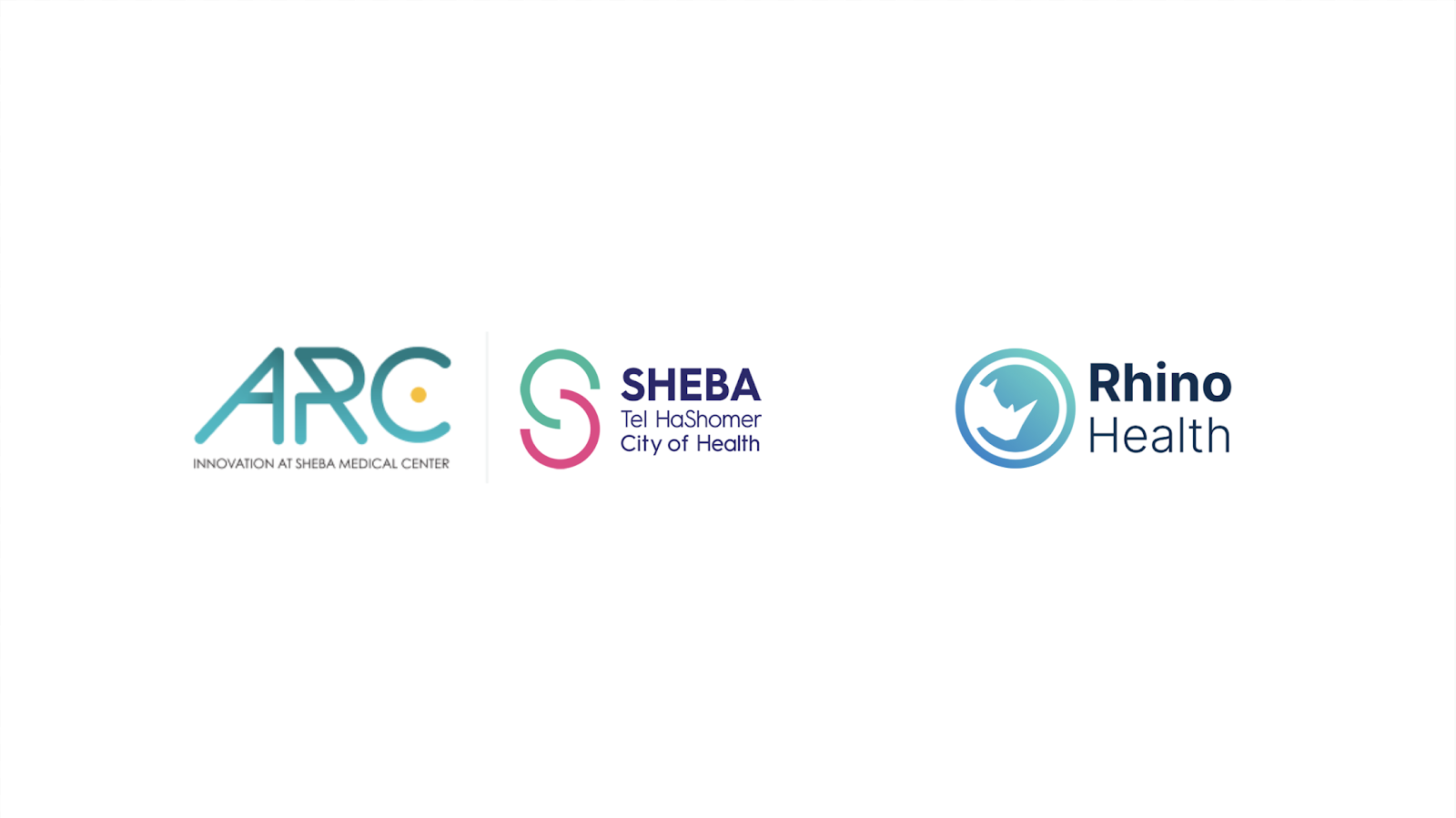
Automating Clinical Data Standardization at for ARC Innovation at Sheba Medical Center with Rhino Health’s Harmonization Copilot
ARC Innovation at Sheba Medical Center, a leading innovation hub dedicated to developing and implementing processes to redesign healthcare globally, is collaborating with Rhino Health to transform the standardization of clinical data. Sheba Medical Center, Israel’s largest medical center and a Newsweek ranked World’s Best Hospital for the last six years, and Rhino Health are leveraging Generative AI to accelerate Sheba’s leadership in international data collaborations. The initiative addresses the critical challenge of interoperability stemming the diversity and complexity of local clinical data, which ranges from patient records and imaging to laboratory results, necessitating a robust solution for efficient and accurate data integration across various healthcare platforms. The ARC Innovation at Sheba Medical Center digital transformation team faces the universal challenge of standardizing heterogeneous clinical data with internationally recognized standards. Standardization is necessary to enhance patient care, facilitate research, and drive global health innovations. Rhino Health’s Harmonization Copilot uses Generative AI to navigate the intricacies of clinical data standardization through a seamless solution that aligns with international standards, such as OMOP CDM¹ and HL7 FHIR², and specific national requirements, such as Israel's FHIR Core profiles.
Generative AI, particularly Large Language Models (LLMs), plays a crucial role in this transformation by enabling sophisticated data harmonization capabilities. The Harmonization Copilot is an application of the Rhino Federated Computing Platform (Rhino FCP), powered by Generative AI, that simplifies and accelerates the data harmonization process by standardizing clinical data to fit international healthcare data standards. Its deep integration with Rhino FCP accelerates the process of extracting data from various sources, transforming it into a structured and standardized format, tracking data quality, and then loading it into a database or data warehouse for future use. This efficient solution ensures the accuracy and reliability of standardized clinical data, providing a solid foundation for enhanced patient care and advanced research capabilities.
Crafted by expert medical informaticists and machine learning engineers, the Harmonization Copilot uses LLMs to perform the challenging task of syntactic and semantic harmonization. This strategic collaboration enables ARC Innovation at Sheba Medical Center to further engage in the data innovation ecosystem. It eliminates the need for extensive vendor contracting and reduces overburdened in-house data engineering and clinical informatics teams, accelerating ARC Innovation at Sheba Medical Center journey toward data-driven healthcare excellence.
“Overseeing our partnership with Rhino Health has been transformative. The Harmonization Copilot has changed how we handle clinical data, seamlessly integrating and standardizing vast arrays of information across multiple systems. The Rhino Federated Computing Platform’s Harmonization Copilot not only enhances our operational efficiency but also boosts our capabilities in patient care and clinical research, strengthening our healthcare innovation assets at ARC Innovation at Sheba Medical Center.”—Benny Ben Lulu, Chief Digital Transformation Officer, Sheba Medical Center and Chief Technology Officer at ARC Innovation.
The road to effective clinical data management
One of the most pressing challenges worldwide healthcare systems face is the harmonization of clinical data to international standards. This complexity arises from many factors, including the need for consistent standards across the healthcare industry. The presence of multiple, often competing standards for data representation and encoding creates a landscape of incompatible formats, which impedes the efficient exchange of crucial health information. Compounding this issue is the reliance of many healthcare institutions on legacy systems. These outdated systems, often embedded with custom data structures, make migration to newer, standardized formats a daunting and resource-intensive task.
Moreover, the heterogeneity of clinical data adds another layer of complexity to the standardization process. Clinical data encompasses diverse formats, from textual notes and laboratory findings to images and diagnostic codes. This diversity poses significant challenges in achieving a uniform standard for data representation. Additionally, there is a critical need to balance the imperative of data sharing with patient privacy. This necessitates sophisticated measures in anonymization, encryption, and access control, further complicating data standardization. In addition, the financial and technical resources required for implementing new data standards often act as a barrier, especially for smaller healthcare systems with limited budgets.
Despite these daunting challenges, the importance of data standardization in the healthcare sector cannot be overstated. Standardized data is a cornerstone for improved patient care, enabling more coordinated care across various providers and institutions. It plays a vital role in reducing medical errors and facilitates the adoption of personalized medicine approaches tailored to individual patient needs. Additionally, large, standardized datasets are invaluable for medical research, accelerating the pace of scientific discoveries and the development of new treatments. From a public health perspective, standardized data is essential for effectively monitoring disease outbreaks, understanding population health trends, and identifying health risks. Moreover, streamlined data exchange can significantly improve administrative efficiency within healthcare systems, reducing paperwork and optimizing resource allocation.
In addressing the challenges of data standardization, recent advancements in LLMs offer a breakthrough solution. LLMs have successfully transformed large volumes of unstructured clinical text into standardized formats. These models go beyond more than just data analysis; they can generate and even execute code, paving the way for the development of autonomous ETL³ agents. This capability represents a significant advancement in the field, allowing for more efficient and accurate processing of complex clinical data. When integrated with The Rhino Federated Computing Platform, these technologies enhance secure and decentralized data collaboration across different healthcare institutions, thus leveraging the principles of Federated Learning.
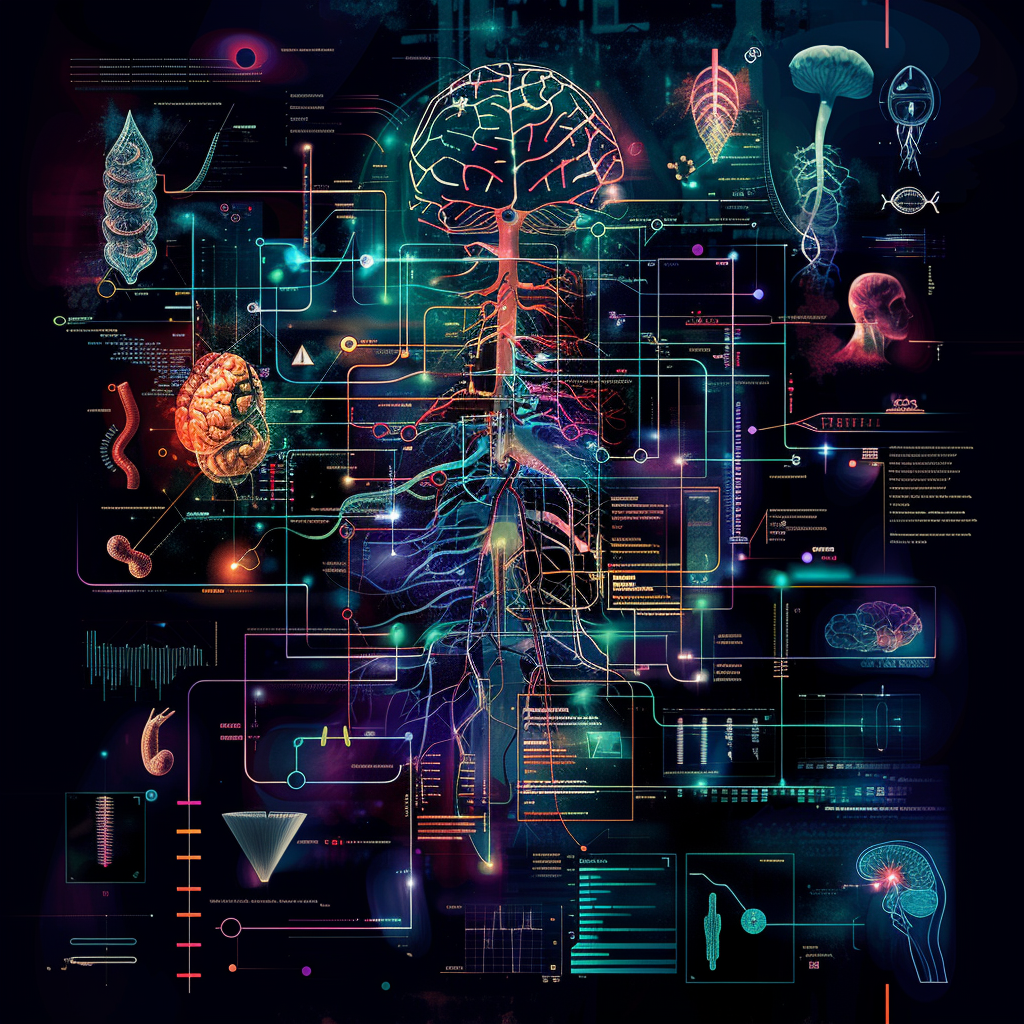
Building the Rhino Federated Computing Platform’s Harmonization Copilot
The Rhino Federated Computing Platform’s Harmonization Copilot addresses the core challenge of data heterogeneity. This solution transforms diverse clinical data into standardized formats and seamlessly integrates into existing healthcare systems, bridging the gap between modern technology and legacy infrastructures. The software is designed to interface efficiently with various legacy systems, adapting to their unique data structures without necessitating extensive overhauls. A standout feature of the Harmonization Copilot is its Retrieval-Augmented Generation (RAG) integration. This crucial advancement effectively transcends the limitations of LLMs, particularly the issue of hallucination.
Regarding data structure adaptability, the Harmonization Copilot showcases remarkable flexibility. Whether dealing with structured data like EHR (Electronic Health Records) or more complex, unstructured data like free-text clinical notes, the Harmonization Copilot employs advanced algorithms to accurately interpret and transform this information into globally recognized formats like OMOP CDM¹ and HL7 FHIR². This adaptability is crucial in accommodating the diverse data representation styles prevalent in different healthcare systems, making the software a versatile tool for data standardization.
Moreover, the software’s integration capabilities extend to country-specific standards like Israel’s CoreIL FHIR profiles, demonstrating its potential for multilingual harmonization to lower barriers to international cooperation. By aligning with global and national standards, the Harmonization Copilot enhances data interoperability within individual healthcare systems and across different geographic and regulatory landscapes. This wide-ranging compatibility positions Rhino FCP’s Harmonization Copilot as a critical player in the global movement towards more interconnected, standardized healthcare data practices.
Behind this sophisticated technology is a team of expert medical informaticists and machine learning engineers who have employed the latest advancements in LLMs to create a system that simplifies and streamlines data standardization. This collaboration of AI technology and deep healthcare expertise ensures that the Harmonization Copilot is not just a tool for data conversion but a comprehensive solution for enhancing the quality and usability of clinical data across the healthcare industry.
Conclusion
By harnessing the power of AI through the Harmonization Copilot, the partnership between ARC Innovation at Sheba Medical Center and Rhino Health is setting new standards in clinical data standardization and interoperability. As we look to the future, the role of AI in healthcare continues to expand, promising more efficient, accurate, and collaborative approaches to patient care and medical research.
Whether you’re a healthcare institution facing the challenge of data heterogeneity, a professional society or a consortium seeking to improve the data quality and usability of your members, or a life sciences company trying to build reusable pipelines with data partners, Rhino Federated Computing Platform (Rhino FCP) Harmonization Copilot can help you. Harmonization Copilot standardizes diverse data formats ensuring interoperability across healthcare systems. Don’t let the complexities of clinical data standardization hold back your potential. Reach out to us today and discover how Harmonization Copilot can benefit you!
About ARC Innovation
ARC Innovation, founded in 2019, is driving the redesign of global healthcare. ARC has developed a structured approach to innovation to equip all parts of the health ecosystem with the tools and resources to advance health care delivery and improve outcomes. With a continuous pipeline of digital health innovation, access to global health leaders and investors and a proven approach to implementing health innovation, ARC is unlocking a sustainable future for global healthcare.
For more information, visit: https://arcinnovation.org/
Notes:
¹ OMOP CDM: Observational Medical Outcomes Partnership Common Data Model is a standardized data model developed to analyze diverse healthcare databases systematically. The model standardizes the structure and format of healthcare data, including patient demographics, clinical events, prescriptions, and measurements, among others. This standardization facilitates aggregating, analyzing, and comparing observational healthcare data from different sources, enhancing research and decision-making in healthcare.
² HL7 FHIR: Health Level Seven Fast Healthcare Interoperability Resources is a standard for exchanging healthcare information electronically. It defines how healthcare information can be formatted and communicated between different systems, ensuring that the data is both interpretable and usable across various healthcare platforms. FHIR supports various healthcare administrative, clinical, and infrastructural data needs, including patient records, billing, care planning, and clinical research.
³ ETL: The process of “Extracting” clinical data from various healthcare systems, “Transforming” this data into a standardized format that aligns with international healthcare data standards, and “Loading” the harmonized data into a centralized repository. This procedure ensures seamless data integration and interoperability across different healthcare platforms, facilitating improved patient care, research, and global health innovations. Utilizing advanced AI technologies, the ETL process overcomes the challenges of data heterogeneity and legacy system integration, enabling healthcare institutions to participate effectively in the data innovation economy.