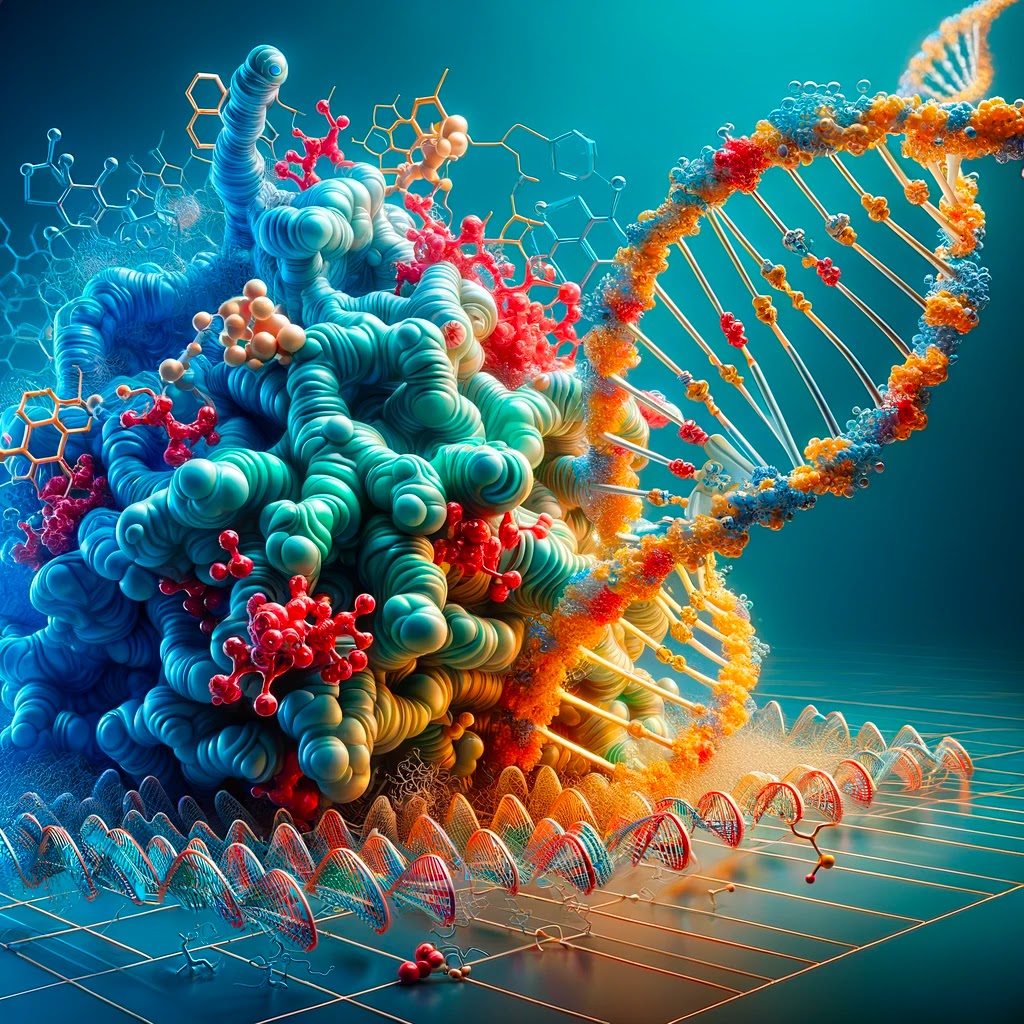
Generative AI for Drug Design with Rhino Health’s Federated Computing Platform
A top 10 global pharmaceutical company recently used the Rhino Health Federated Computing Platform (FCP) for a novel application of generative AI (GenAI) for drug design.
Rhino Health is excited about the potential of GenAI in healthcare & life sciences. This case study is an example of applications for protein engineering. GenAI can aid in protein structure prediction: deep learning models can predict protein sequences’ folding patterns, which helps researchers design protein modifications such as improving stability. GenAI can also aid researchers in protein sequence generation - creating entirely new sequences with the researcher’s desired functionality or structural properties such as binding to specific targets. This emerging application of GenAI will help to unlock innovation while avoiding the legacy paradigm of time-consuming trial & error. Rhino’s partner NVIDIA are currently using their BioNeMo product to deliver cloud-based GenAI, and contributed to this instance of adapting the model for their FLARE framework.
In this case specifically, our client is going one step further: pioneering the application of Federated Learning (FL) to GenAI. Our client was interested in FL as a method of establishing a consortium with a combination of peers and academia.data. Typically, this type of collaboration can easily take a year to establish - and is often limited by data sharing concerns. Some imperfect solutions are provided either via locally supported ‘data enclaves’ or more advanced ‘clean rooms’ with limited MLOps capabilities and no workflow for seamless processing of data from multiple sources. Rhino Health was able to streamline this process - installing FCP edge nodes in just days from our introduction, and with a platform that doesn’t compromise on the subsequent utility of the data.
The client was also concerned with protecting its intellectual property when collaborating with third parties. The Rhino FCP’s model security features gave our client the confidence needed to work with a consortium of collaborators. The Rhino FCP secures both model code / architecture and model weights / parameters. We use strict role-based permissioning to control who has access to model code and parameters. We also allow developers to encrypt their models & parameters (including supporting homomorphic encryption) to avoid even Rhino Health being able to access them.
The Rhino FCP allows for data scientists to complete their end-to-end workflow all in one place - from data pre-processing to experiment management, all using the same set of tools with which they are already familiar (e.g. Jupyter Notebooks, TensorBoard). The Rhino FCP enables users to track computations across the consortium in real time - valuable when coordinating intensive workloads with multiple partners.
Our client successfully completed this project in phases by meeting Business and Scientific KPI's and requirements. This project began by using DiffDock, an open source diffusion generative model (DGM) designed for molecular docking, before moving on to proprietary IP. The client wanted to first evaluate Rhino’s ability to build a network with partners, then to see the power of FL to improve model results, and last to make sure they were comfortable that we could meet their security requirements while offering a smooth workflow. Ultimately, FL did indeed generate strong improvement in binding site predictions. We are proud to have met each of these criteria, and to be supporting their ongoing innovative research.
To learn more about using the Rhino Federated Computing Platform for generative AI or other drug design applications, contact us.