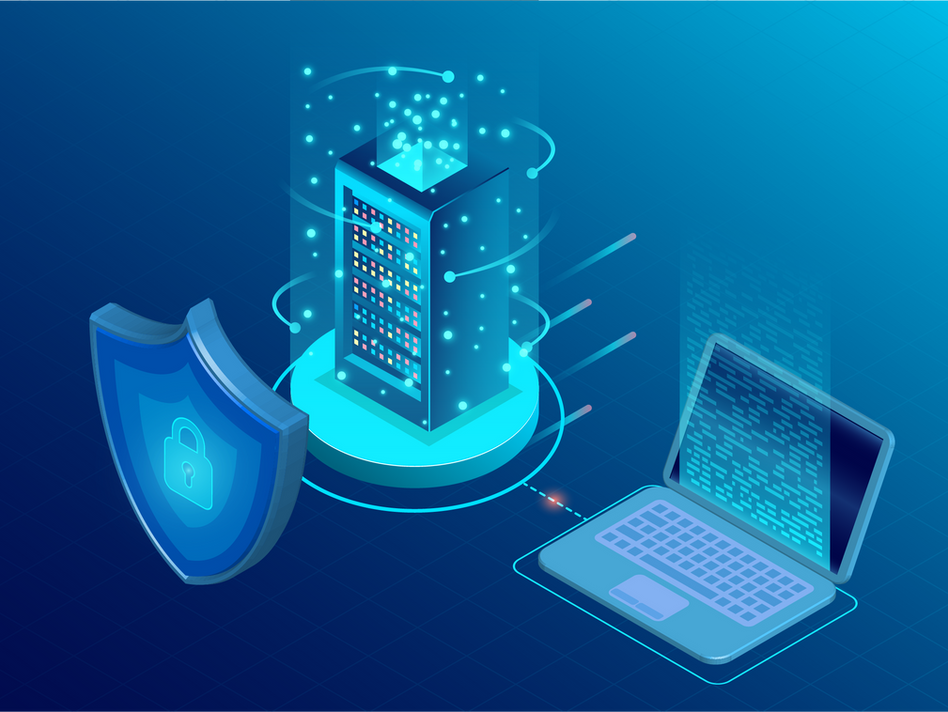
Why Assuta Medical Centers, a Leading Israeli Health Network, Chose to Partner With Rhino Health
Tackling the increasingly complex challenge of utilizing health data without creating risk by transferring it. Federated Learning Can help.
Today’s Reality: A surge in data-sharing requests demands re-thinking the current approach:
Hospitals sit on stockpiles of healthcare data, exhibiting what people are hospitalized with, how they were treated, and associated clinical outcomes. When these datasets are combined and compared, they provide hugely valuable insights that could advance medical research and drug development. One of the key factors here is the ability to access and assess diverse data - gathered from different patient populations in different places over time.
There are clear benefits in sharing health data: researchers can learn what treatments are best for people with certain medical conditions; startups and global medical companies can use data to develop algorithms assisting in diagnosing and managing care. That being the case, hospitals are overwhelmed by what seems to be a lucrative new opportunity – the co-development of data-driven solutions with tech companies. The number of tech startups and big tech players aiming to bring AI/ML solutions to healthcare and pitching hospitals on data-sharing partnerships is skyrocketing.
“Every week, we get approached by multiple companies that wish to participate in research projects and provide access to our data for supporting innovative endeavors with the potential to improve patient outcomes. It’s exciting, but it’s also overwhelming. The reality is that managing many data-sharing agreements with different partners can create an untenable IT, operational, and administrative burden - and could put us at risk of violating privacy regulations,” said Daniel Rabina, Director of Innovation and Ventures Development at Assuta Medical Centers.
What’s Required: Utilizing health data within ever-evolving regulatory frameworks
As opportunities to advance healthcare solutions abound - fueled by the increase in health data created around the world - so do the complexities of utilizing this data. Ethical and legal frameworks are rapidly evolving to address this reality. HIPAA, GDPR, and other country-specific regulations regarding the use of health data are intended to help guide the work of research use committees. These legal frameworks strive to balance the desire to encourage and promote research collaborations and medical innovation with the need to protect patient privacy. For example, a government-defined process of de-identification - by which personally identifying information is removed from the health data - mitigates privacy risks to individuals and supports the secondary use of health data for comparative effectiveness studies, policy assessment, life sciences research, and other endeavors.
But de-identifying data does not eliminate all the potential risks of transferring the data. If that data is later combined with data from other sources, re-identification can occur. Inappropriate cross-referencing of data with supplemental elements may lead to this unintended consequence - which directly violates a second key requirement of HIPAA that specifies the need to minimize the risk of re-identification.
Any time data is licensed and transferred outside the walls of the medical institution where it was created; the institution loses control over what will happen next with these files - including an increased likelihood the data may be re-identified. Within the context of a different environment - with varying postures of security, different access controls - that data is now subject to new risks. The other party may violate contractual obligations to protect it. And, of course, this data - which contains sensitive personal and business information - is a prime target for hackers, which can lead to identity theft, financial fraud, malignant manipulations, or target advertisement.
The harsh truth: once data is outside the walls of the medical institution, it is out. Despite all the legal provisions and well-intended data-sharing agreements, the data owner actually has zero control over it. Realizing this, many institutions are increasingly reluctant to participate in data-sharing and data-licensing agreements, which - unfortunately - stymies medical advancements and slows technical innovation.
The Federated Opportunity: Distributed access without transferring control over data
The vexing question facing medical institutions: Is it even possible to advance health research and ensure patient privacy and strike the right risk/reward balance for the institution? In short, yes.
Forward-thinking institutions are starting to explore strategies to avoid transferring actual data, such as federated learning. Federated Learning (FL) is a machine-learning technique that trains an algorithm across multiple servers at different hospitals, keeping health data samples within an institution behind the firewall. Only the AI model characteristics (e.g., weights, parameters, gradients) are shared. Then the characteristics are combined in the form of a consensus model, gaining insights collaboratively. Successful implementation of FL holds significant potential for facilitating precision medicine at a large scale, resulting in models that produce unbiased decisions, optimally reflect an individual's biology, and account for governance and privacy concerns.
Rhino Health has developed a turnkey software that turns Federated Learning into a comprehensive "Federated Learning As A Platform" solution for healthcare. The platform allows model developers to securely collaborate on projects using distributed data - without ever moving it or exchanging control. Democratizing multi-institutional collaborations that utilize data from larger, more diverse real-world patient populations results in the development of more robust and generalizable AI solutions. Using a combination of Distributed Computation with Federated Learning eliminates the need to export any data outside the originating institution’s IT environment. In addition, the Rhino Health platform can serve as a secure tool for tracking model performance over time in clinically relevant situations and enabling re-training as needed while leveraging both data acquired prospectively and historical cohorts, all without compromising data lineage and traceability.
Assuta Medical Centers, a leading health system in Israel, has recently joined the growing global network of hospitals adopting the Rhino Health platform to make their health data accessible to industry innovators while safeguarding patients' rights and setting a new privacy standard.
“With federated learning, made possible by Rhino Health, we do not have to make a trade-off between participating in research collaborations that will ultimately improve patient outcomes and protecting the privacy of our patients and the integrity of our institution. Realizing the benefits of sharing data insights without ever actually sharing the data itself is a game-changer. As we think about the future of R&D in healthcare, we already see how Rhino’s network transforms data collaborations between major hospitals around the globe. We believe it is going to catalyze R&D partnerships and will accelerate the development and validation of new and unexpected clinical data-driven tools.” Said Dr. Michal Guindy, Head of Ventures and Innovation and Head of Medical Imaging at Assuta Medical Centers.
Rhino Health invites health tech startups and other life sciences companies to join this movement and take advantage of this privacy-first federated approach. Protect patient privacy and promote diversity and equitability in healthcare by gaining access to large and diverse datasets through Rhino Health's growing global network of AI developers and industry innovators.